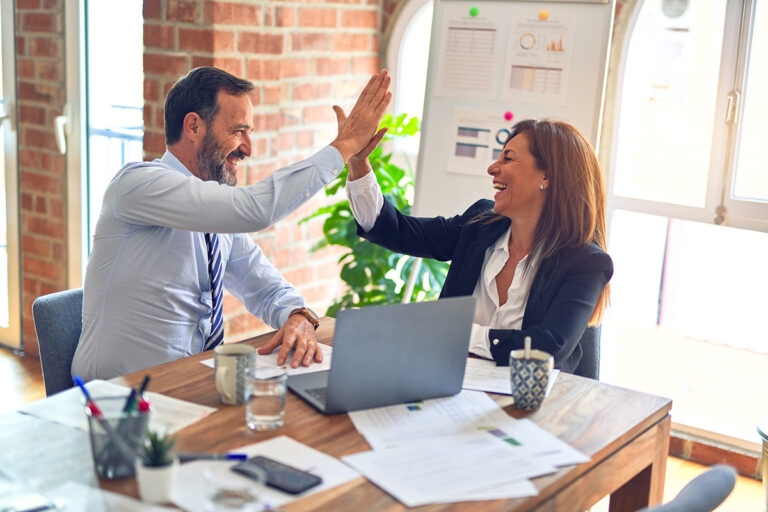
DP-100: Designing and Implementing a Data Science Solution on Azure (3 pv)
1800 euroa /hlö + alv
11.-13.05.2020, Tieturi Oy, Helsinki
1800 euroa /hlö + alv
11.-13.05.2020, Tieturi Oy, Helsinki
Koulutuksessa opit käyttämään Microsoft Azuren Machine Learning PaaS-työkalua sekä sen tekoäly- ja koneoppimisominaisuuksia. Koulutuksen jälkeen osaat hallinnoida datan siirtoa ja prosessointia analysointia varten.
Koulutus valmistaa sertifiointitestiin DP-100: Designing and Implementing a Data Science Solution on Azure.
Kenelle?
Koulutus soveltuu data-alan ammattilaisille, jotka haluavat toteuttaa ja käyttää koneoppimisratkaisuja pilvessä ja joilla on jo kokemusta Pythonista ja koneoppimisen ohjelmista, esimerkiksi Scikit-Learnista, PyTorchista ja Tensorflowsta.
Esitiedot
Esitietovaatimuksina on:
– perustiedot Microsoft Azuresta
– kokemusta Python-ohjelmointikielestä sekä NumPy-, Pandas- tai Matplotlib-kirjastoista
– datatieteen perustiedot ja mm. kokemusta koneoppimisen mallien kouluttamisesta esimerkiksi Scikit-Learnillä, PyTorchilla tai Tensorflowla.
Koulutuspäivät alkavat klo 9.00 ja loppuvat noin klo 16.-16.30. Aamiaista on tarjolla klo 8.15 lähtien.
Sisältö:Module 1: Introduction to Azure Machine Learning
In this module, you will learn how to provision an Azure Machine Learning workspace and use it to manage machine learning assets such as data, compute, model training code, logged metrics, and trained models. You will learn how to use the web-based Azure Machine Learning studio interface as well as the Azure Machine Learning SDK and developer tools like Visual Studio Code and Jupyter Notebooks to work with the assets in your workspace.
LessonsGetting Started with Azure Machine Learning
Azure Machine Learning Tools
Lab : Creating an Azure Machine Learning Workspace
Lab : Working with Azure Machine Learning Tools
After completing this module, you will be able to:
– Provision an Azure Machine Learning workspace
– Use tools and code to work with Azure Machine Learning
This module introduces the Designer tool, a drag and drop interface for creating machine learning models without writing any code. You will learn how to create a training pipeline that encapsulates data preparation and model training, and then convert that training pipeline to an inference pipeline that can be used to predict values from new data, before finally deploying the inference pipeline as a service for client applications to consume.
LessonsTraining Models with Designer
Publishing Models with Designer
Lab : Creating a Training Pipeline with the Azure ML Designer
Lab : Deploying a Service with the Azure ML Designer
After completing this module, you will be able to:
– Use designer to train a machine learning model
– Deploy a Designer pipeline as a service
In this module, you will get started with experiments that encapsulate data processing and model training code, and use them to train machine learning models.
LessonsIntroduction to Experiments
Training and Registering Models
Lab : Running Experiments
Lab : Training and Registering Models
After completing this module, you will be able to:
– Run code-based experiments in an Azure Machine Learning workspace
– Train and register machine learning models
Data is a fundamental element in any machine learning workload, so in this module, you will learn how to create and manage datastores and datasets in an Azure Machine Learning workspace, and how to use them in model training experiments.
LessonsWorking with Datastores
Working with Datasets
Lab : Working with Datastores
Lab : Working with Datasets
After completing this module, you will be able to:
– Create and consume datastores
– Create and consume datasets
One of the key benefits of the cloud is the ability to leverage compute resources on demand, and use them to scale machine learning processes to an extent that would be infeasible on your own hardware. In this module, you’ll learn how to manage experiment environments that ensure consistent runtime consistency for experiments, and how to create and use compute targets for experiment runs.
Lessons
Working with Environments
Working with Compute Targets
Lab : Working with Environments
Lab : Working with Compute Targets
After completing this module, you will be able to:
– Create and use environments
– Create and use compute targets
Now that you understand the basics of running workloads as experiments that leverage data assets and compute resources, it’s time to learn how to orchestrate these workloads as pipelines of connected steps. Pipelines are key to implementing an effective Machine Learning Operationalization (ML Ops) solution in Azure, so you’ll explore how to define and run them in this module.
LessonsIntroduction to Pipelines
Publishing and Running Pipelines
Lab : Creating a Pipeline
Lab : Publishing a Pipeline
After completing this module, you will be able to:
– Create pipelines to automate machine learning workflows
– Publish and run pipeline services
Models are designed to help decision making through predictions, so they’re only useful when deployed and available for an application to consume. In this module learn how to deploy models for real-time inferencing, and for batch inferencing.
LessonsReal-time Inferencing
Batch Inferencing
Lab : Creating a Real-time Inferencing Service
Lab : Creating a Batch Inferencing Service
After completing this module, you will be able to:
– Publish a model as a real-time inference service
– Publish a model as a batch inference service
By this stage of the course, you’ve learned the end-to-end process for training, deploying, and consuming machine learning models; but how do you ensure your model produces the best predictive outputs for your data? In this module, you’ll explore how you can use hyperparameter tuning and automated machine learning to take advantage of cloud-scale compute and find the best model for your data.
LessonsHyperparameter Tuning
Automated Machine Learning
Lab : Tuning Hyperparameters
Lab : Using Automated Machine Learning
After completing this module, you will be able to:
– Optimize hyperparameters for model training
– Use automated machine learning to find the optimal model for your data
Many of the decisions made by organizations and automated systems today are based on predictions made by machine learning models. It’s increasingly important to be able to understand the factors that influence the predictions made by a model, and to be able to determine any unintended biases in the model’s behavior. This module describes how you can interpret models to explain how feature importance determines their predictions.
LessonsIntroduction to Model Interpretation
using Model Explainers
Lab : Reviewing Automated Machine Learning Explanations
Lab : Interpreting Models
After completing this module, you will be able to:
– Generate model explanations with automated machine learning
– Use explainers to interpret machine learning models
After a model has been deployed, it’s important to understand how the model is being used in production, and to detect any degradation in its effectiveness due to data drift. This module describes techniques for monitoring models and their data.
LessonsMonitoring Models with Application Insights
Monitoring Data Drift
Lab : Monitoring a Model with Application Insights
Lab : Monitoring Data Drift
After completing this module, you will be able to:
– Use Application Insights to monitor a published model
– Monitor data drift
Koulutuspaikka:
Tieturi Oy, Mannerheimintie 15, Helsinki
Voit osallistua koulutukseen myös etänä.
Ajankohta:
11.-13.05.2020
Hinta:
1 800 euroa /hlö + alv 24 %.
– koulutuksen voi maksaa Microsoftin SA-koulutusseteleillä, tarkista tarvittavien seteleiden määrä info@tieturi.fi.
– maksaessasi koulutuskortilla veloitamme sinulta materiaalimaksun 160 euroa.
Ilmoittautumisen peruutusehdot:
Alle 14 päivää ennen koulutusta tehdyistä perumisista perimme osallistumismaksusta 50 %. Alle 7 päivää ennen koulutusta tehdyistä perumisista perimme osallistumismaksun täysimääräisenä. Mikäli et saavu koulutukseen, veloitamme koko osallistumismaksun ja mahdolliset jo tilatut testit.
Pidätämme oikeuden muutoksiin kansainvälisten koulutuskumppaniemme koulutuksiin. Näiden koulutusten osalta olemme sinuun yhteydessä ilmoittautumisesi jälkeen.
DP-100: Designing and Implementing a Data Science Solution on Azure (3 pv)
1800 euroa /hlö + alv
11.-13.05.2020, Tieturi Oy, Helsinki